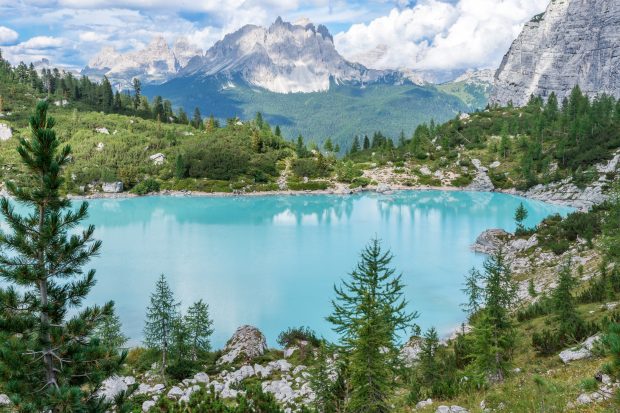
For decades, organisations have been obsessed with technology. First with machines that could make production faster, cheaper and more efficient. Then systems that could manage the information created by these machines, which increased as the commoditisation of business and personal computers advanced. Information Technology systems increased in complexity, and as computer systems began to be networked, the need to formally design IT systems became evident. A new breed of designers started to appear to focus on the various aspects of the ever increasing technology landscape. It became inconceivable to try to solve complex IT problems without appropriate design, because the variability of the components of the system was just too great. This of course became more acute as organisations interacted and merged, many of them with systems based on various technologies, some of which were already becoming legacy but couldn’t just be replaced. And so the era of enterprise, technical and solution architects, user researchers, business analysts, etc, begun.
From data warehouses to data lakes
Information Technology thrived through the last quarter of the 20th century. Personalised services and devices became pervasive, and so did the need to store the information collected by them, which in turn powered new services and business models. Database servers became data warehouses, and information management turned not only into a storage problem, but also a processing challenge. New paradigms of storing and processing data were born, and data warehouses became data lakes, and rigid parallel computing systems evolved into flexible distributed computing platforms, powered by virtual machines, containerization and high speed internet.
By now organisations had understood that they were sitting on masses of data generated by their own operations, and that new technology and data management systems gave them the tools to extract insights that could help their businesses. While some turned to ready-made Business Intelligence software, others began to explore what could be done by programming new processing pipelines within their business models, or even creating new businesses by exploiting new data generated by a new breed of online services. And so data analysts gave way to data scientists, data engineers, and this is pretty much where we find ourselves at the moment.
Where we are now
The same way the IT revolution was powered by design to cope with the complexity of the technology landscape, you would think that the data revolution should follow suit. In the end, data is being generated by technology, and so it is as varied as the technology itself. Instead, however, we see that the data revolution is not being powered by design but by analysis, which is a product of data. This is like creating IT services without planning how the infrastructure should support them: for each service to be provided, the technology infrastructure is put in place to deliver it. Clearly, this would become unsustainable for organisations with multiple services which need to run on top of shared IT infrastructure. Organisations want to optimise investment, and not buy new equipment, lay new cables or install new software for each problems they are trying to resolve.
So why are we not designing data solutions the same way we design IT solutions? The answer is quite complex and probably a combination of multiple factors. Firstly, data is immaterial and it doesn't cost money in such an apparent way as IT. So the inefficiencies of data analysis processes do not compute in the balance sheets in the same way as IT does. Secondly, exploiting their data assets and making data profitable are new concepts for many organisations. Many leaders know they need to do something with their data wealth but data analysis / science is a bit of a mysterious art to them. Thirdly, organisations know IT well, and when faced with a problem that sounds like technology they try to solve it throwing more technology at it i.e. buy a cloud service, install a data lake or a distributed platform or just hire more people. Finally, some organisations struggle with defining what a design solution for data might be. It is easy to visualise connecting physical systems together, and conceptually, it is easier to see that certain technology glue must be required to join up services, such as front end applications with backend servers. But what are you trying to connect with data? What is the glue that makes products based on data more efficient?
Why Data Architecture?
Based on all this, the answer to this last question is unsurprisingly design or, following the IT parlance, architecture. Data Architecture, to be more precise. And Data Architecture is the most overlooked piece of the puzzle for organisations to take full advantage of their data estates. Architecting the data allows organisations to build one shared design infrastructure so that data consumers can understand what data they have, where it is and what it means. It allows organisations to manage their data estate, which should be separate from, but related to, the organisation's technology and analysis strategy. Organisations that skip the data architecture step find themselves repeating the same data pre-processing steps for every application they create. Their data scientists spend more than half their time wrangling data from various parts of the company, trying to understand what variables in different data sets mean, cleaning them and transform them into something they can use. And while data wrangling is a necessary step of data exploration, it should not take most of the time of the data analysis process and it most definitely should not be part of the production process.
Data architecture doesn’t just help organisations be more efficient and therefore expedite the development of data applications, but is a key factor on data quality, and thus on the final products themselves. Resolving data inconsistencies and errors shouldn’t depend on the experts that build the analysis pipeline. Those analyst should be provided with datasets that have been produced and described following agreed standards, and adherence to those standards has been governed appropriately throughout the data lifecycle. Data standards and governance must be an organisation-wide priority, because errors at source can creep all the way up to the analysis pipeline and end up in product outputs. Not to mention that appropriate governance is part of the legal obligations of organisations regarding the management of their data.
Where next?
So if you are a leader of an organisation undertaking a digital transformation and planning to take seriously the potential of your data estate, do not just buy a cloud solution and hire data scientists. You will need that, but you will also need to know what data you have, how to make sense of it and how to manage it, and only data architects will give you that.
Leave a comment